DREAM: Data-driven Reliability-centred Evolutionary Automated Maintenance for Offshore Wind Farms
​
A research project funded by EDF Energy R&D Centre London
Overview
​
The costs of Operation and Maintenance (O&M) in offshore wind farms can be significantly reduced through condition monitoring, data analytics and intelligent maintenance planning. This can be achieved via combining optimization algorithms, fault prognosis and reliability models. DREAM is focusing on the development of bio-inspired optimisation techniques that exploit fault prognosis to continually produce and update an evolving optimal plan of O&M. This plan explores the enormous design space of possible dynamic maintenance schedules to generate optimal maintenance plans that optionally take into account logistics and weather, and optimise reliability and energy profiles whilst minimising maintenance costs and reducing downtime. An overview of the DREAM framework is illustrated below
​
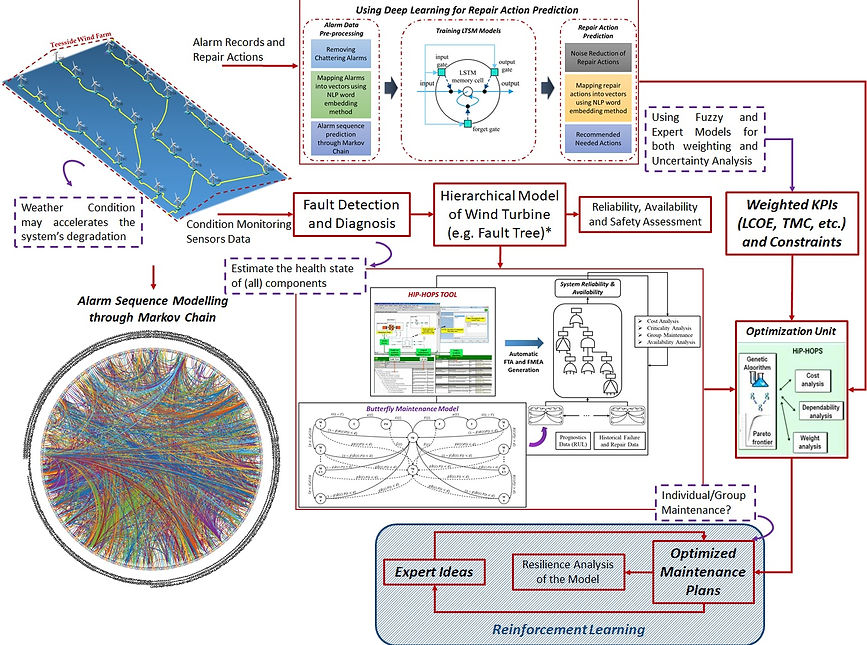
The framework involves four main steps.
​
-
Condition monitoring data is used for estimation of Remaining Useful Life (RUL) from which expected failure distributions are projected and fed to a Semi-Markov Process (SMP) within HIP-HOPS, an automated reliability estimation tool, in order to project reliability and availability in real time.
-
Using natural language processing and deep learning, a system is built through which possible maintenance procedures and its requirement are recommended.
-
An evolutionary optimization algorithm uses estimates of reliability and possible maintenance actions to continually generate and update an optimized maintenance plan for an offshore wind farm.
-
In the context of reinforcement learning, the maintenance plan is judged by experts and is adjusted in subsequent iterations.
The case study of this research is on the Teesside offshore wind farm known as Redcar and owned by EDF Energy. Information such as alarm data, repair plans, maintenance procedures, etc. related to the Teesside Wind Farm is used in this research. This offshore wind turbine has twenty seven 2-3 MW turbines that can guarantee the total capacity of 62 MW. The following figure shows the wind farm's layout and the list of the available data.

Overall the project has three very practical objectives which we feel can benefit industry:
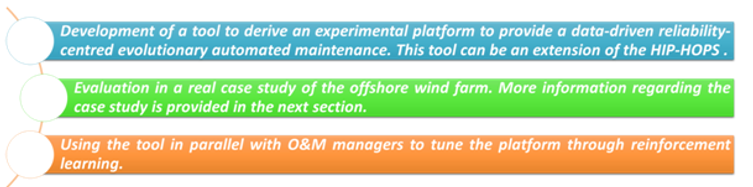
Early results in the area of alarm management
​
In an Offshore Wind Farm, the SCADA alarm system informs decisions about maintenance. As part of DREAM, we investigate an advanced system that could link alarms to advice on repair actions. A result in this area is a Markov chain of alarm sequences created from historical data for the Teesside Offshore Wind Farm. From this Markov chain both prognostic and diagnostic information can be generated: for instance given an alarm, the list of most probable alarm sequences can be projected; on the other hand, given an incomplete alarm sequence missing elements could be estimated pointing better towards sources of problems.
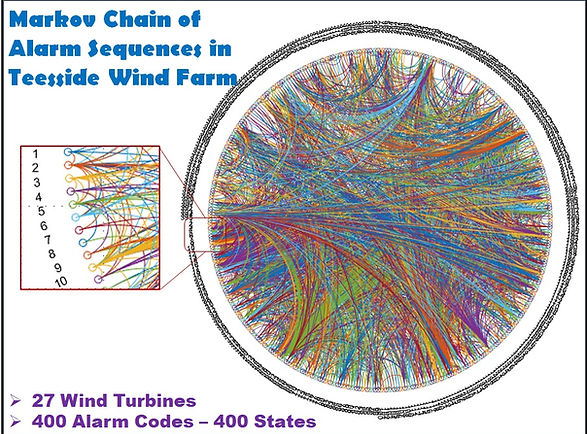
Further information
​
DREAM is undertaken within the Dependable Intelligent Systems group led by Professor Papadopoulos, while the main contributor is Mr Koorosh Aslansefat. The group pioneers internationally methods and tools for design of dependable systems. Tools include HiP-HOPS and Safety Designer, the latter together with ESI GmbH. We lead new nature-inspired algorithms which imitate the hunting behaviour of Penguins, and have shown that this work applies to the safety of autonomous cars. The BBC has run an article on "Hungry Penguins Keep Car Code Safe". Our tools have been taken up by several major corporations which include Toyota, Volvo, Honda, Embraer and Électricité de France (EDF).
​
Sponsors
​
EDF Energy R&D UK Centre is sponsoring the DREAM research project and EDF Renewables UK providing access to the Teesside Offshore Wind Farm data.
​
Main EDF Contact: Dr Tariq Dawoo, Principal Engineer Asset Integrity Management for Nuclear & Offshore Wind, EDF (UK)
​
External Collaborators
​
Our external collaborations include the Federal University of Juiz de Fora in Brazil, where Dr André Luiz de Oliveira and his team are pursuing work on variability and product lines within the scope of the DREAM project. Contributions include on model-based safety assessment of product lines and safety of machine learning applications in the energy domain
​